Imagine asking a sophisticated AI model for tips on making the perfect pizza, only to be told to use glue to help the cheese stick. Or watching it fumble through basic arithmetic problems that a middle schooler could solve with ease. These are the limitations and quirks of generative AI and the large language models (LLMs) that underpin them. They’re happening because AI models are running out of good training data, causing them to plateau.
This is a cycle in innovation that repeats throughout history: For a long time, an almost undetectable amount of knowledge and craft builds up around an idea, like an invisible gas. Then, a spark. An explosion of innovation ensues but, of course, eventually stabilizes. This pattern is called an S-Curve. For example:
- TCP/IP: Originating in ideas from the 1960s, TCP/IP saw significant acceleration after its 1974 RFC and stabilized with version 4 in 1981, which still underpins the modern Internet.
- The Browser Wars: During the late 1990s, web browsers rapidly evolved into interactive, programmable platforms. Since then, improvements have largely been incremental.
- Mobile Apps: The iPhone App Store’s launch in 2008 spurred a surge in mobile app innovation. Today, truly novel mobile apps are rare.
The AI plateau
The AI revolution is following this curve. In a 1950 paper, Alan Turing was one of the first computer scientists to explore how to build a thinking machine, starting the slow buildup of knowledge. Seventy years later, the spark: A 2017 research paper, Attention Is All You Need, leads to OpenAI’s development of ChatGPT, which convincingly mimics human conversation, unleashing a global shock wave of innovation based upon generative AI technology.
For a while, each subsequent LLM release, and releases from other companies like Anthropic, Google, and Meta, offered drastic improvements.
But lately, progress has ebbed. Consider this chart of performance increases of OpenAI’s flagship model:
Although every benchmarking system has shortcomings, clearly the pace of change is no longer setting the world on fire.
Lack of good training data is what has caused AI capabilities to plateau, and access to the next frontier of data is what AI needs to make the jump to the next S-Curve.
Today’s LLMs were primarily trained on publicly available internet data, harvested from Github, Reddit, WordPress, and other website scraping and licensing activities. But it’s no longer enough to sustain model improvements. To fill its insatiable hunger for new data, OpenAI, for instance, developed a neural net called Whisper to transcribe a million hours of YouTube videos for GPT-4. Other novel methods being used include employing human-data labelers via services like ScaleAI (many of which have struggled with negative press for poor working conditions). However, the data clearly shows that continuing down these paths are leading to diminishing returns.
The next curve: Business data
We believe the real breakthrough that will allow humanity to jump to the next S-Curve is data produced at work. Workplace data—e.g. product specifications, sales presentations, and customer support interactions—is of far higher quality than what’s left of public data for training purposes, especially compared to running the dregs of the internet through the transformer mill. (The results of which may be why a lot of AI-generated content is already being called “slop.”)
Startups that unlock and harness business data will create significant value and tools that enterprises actually want to adopt. The potential for AI in the B2B space is vast and largely untapped.
The data is there: Knowledge workers continuously produce business data at an incredible cadence:
- In 2020, Zoom captured 3.3 trillion meeting minutes—55 billion hours—of dynamic human engagement. This dwarfs the estimated 150 million hours of total YouTube content.
- Ironclad processes more than 1 billion documents annually.
- Slack delivers more than a billion messages per week.
So is the value: As a proxy, we compared the average revenue per user (ARPU) of top consumer apps against the per-seat price of select B2B apps. Even the most “consumer-oriented” business apps, such as Notion, still earn much more revenue per user than consumer tech companies:
A slippery slope
That said, enterprises are right to be wary as LLM providers tackle business-use cases (see OpenAI’s Rockset acquisition and Anthropic’s vision for B2B). OpenAI and Anthropic claim that they do not train models on data from business tier subscriptions. History tells us that the pressures of growing their business might force them to backtrack.
For example, Meta long claimed to ignore users’ activity on partner websites while they were logged out. $725 million in privacy-related settlements later, it’s still gobbling up consumer data at a massive rate. As a cloud software pioneer, Salesforce originally committed that all customer data would not be shared with third parties. Its current privacy policy negates this.
This time, the stakes are higher. In the beginning of the cloud era, SaaS applications were primarily used in “noncore processes”—anything absolutely core to a business would be built in-house. But in order to make AI work, businesses need to feed it the essence of their competitive advantage: Everything from knowledge bases to internal processes, contracts, sales calls, and other proprietary, sensitive data.
That’s why we believe businesses themselves, not closed-source AI companies, need ownership and control of their proprietary models.
Just as the New York Times is fighting to protect its IP, businesses should resist the big AI companies’ appetite to harvest their proprietary data in the same manner they sucked up public data.
Four opportunities ahead
The big AI companies are becoming entrenched; 82% of startups in our recent survey are using either OpenAI, Google, or Anthropic to power their AI features. But all is not lost. We have identified four areas of opportunity for new startups to solve the AI plateau in a way that is compatible with the needs and imperatives companies face.
- Engage Experts: High-quality training data comes from field experts, not crowdsourced labelers. Startups should embed themselves in expert communities and explore novel incentive structures like gamification to source data. Companies like Centaur Labs and Turing leverage networks of professionals to improve data quality.
- Leverage Latent Data: A treasure trove of valuable data exists within business apps such as Salesforce and Slack. Startups can help enterprises prepare this data for AI use. Companies like Unstructured and Reducto can help enterprises ingest complex documents for use with LLMs, while tools like LlamaIndex connect data across various applications for AI processing.
- Capture in Context: Businesses generate new data daily. Capturing this data without disrupting workflows is crucial. Apps like Zoominfo and Textio allow organizations to guide workers into doing their jobs more effectively, and make better decisions across the organization.
- Secure the Secret Sauce: Enterprises should build and deploy custom models to stay in control and protect IP. Techniques like federated learning allow model training without sensitive data leaving a user’s device, ensuring privacy. Startups such as Flower and FedML help organizations utilize these techniques.
This is just the tip of the iceberg: There are myriad opportunities to solve the AI plateau, sparking the leap to the next S-Curve in AI performance.
It’s also just the latest chapter in the ongoing story of human technological advancement. AI should be based on human discovery and knowledge and crafted with human-centric attributes of privacy and quality in mind. In the dance of cocreation with AI, humans must always take the lead.
A version of this piece first ran on the Emergence Capital blog.
Login to add comment
Other posts in this group



Flashes, a photo-sharing app that’s linked to X-alternative Bluesky, launc

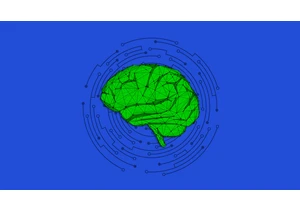

The Brannock device—that sliding metal gadget used in shoe stores to measure the dimensions of your feet—was invented

The generative AI revolution has turned into a global race, with mixtures of mode