Machines outperform humans in countless ways—crunching data, organizing information, finding patterns, and even generating ideas. Yet there’s one crucial skill where we still hold the edge: predicting the future.
In the high-stakes venture capital world, an obscure Sonoma, California, firm is leveraging this human edge to potentially revolutionize the industry. Third Round Analytics Capital (TRAC) combines human expertise with machine learning to spot the most promising startups.
Using insights from “superforecaster” VCs, their 2020 Fund I identified nine potential unicorns among 46 investments, ranking #11 out of 151 comparable funds. Their 2022 Fund II is performing even better, ranking #2 out of 138 comparable funds by early 2024, according to Preqin.
This success builds on a growing trend of human-powered prediction platforms. Since 2014, when professor Philip Tetlock’s groundbreaking study in Science showed that prediction markets and crowdsourced forecasts could outpace intelligence analysts in geopolitical forecasting, these platforms have exploded. Polymarket, the largest U.S. prediction market, is growing at a staggering 200% compound annual growth rate despite regulatory hurdles.
While Tetlock’s work proved some individuals are orders of magnitude better forecasters than others, the financial world was slow to fully exploit these implications. TRAC’s founders saw an opportunity to apply this concept to venture capital, an industry where accurate predictions can lead to outsized returns.
“We realized that if we could identify and track the best-performing VCs—our own set of “superforecasters”—we might be able to predict which startups would succeed,” explains Joe Aaron, one of TRAC’s cofounders.
Despite its name, TRAC focuses on early-stage companies, primarily investing in the first two funding rounds, such as Seed and Series A, where the highest returns are often realized.
Ironically, chance rather than data brought together three individuals with complementary skills and a shared vision. Fred Campbell, a cofounder of CrowdSmart, a pioneering AI-driven investment firm, knew Aaron, a futurist and the cofounder of DaaS Analytics, who in turn knew Dick Fredericks, an investment banker and former diplomat. The three cofounders’ combined expertise in AI, data analytics, and traditional finance laid the groundwork, with Fredericks as its Chairman. Chief data scientist Steve Marek, who brought the technical skills to transform vision into reality, joined as a fourth cofounder.
At its core, TRAC uses a proprietary AI model that analyzes vast amounts of data to identify promising startups and predict their likelihood of success. What sets TRAC apart from other data-driven investment firms (funds like Sequoia Capital and Andreessen Horowitz use data to identify promising startups) is its combination of quantitative analysis with, oddly, a human element, or more precisely, a superhuman element.
TRAC created a database that collects information on early-stage (professional) venture capital investors. “To make our cut, a venture capitalist must be in the top 1/10th of 1% of all early-stage investors,” explains Aaron. “They consistently make a profit on two-thirds of their positions, and one in five of their investments returns over 10X.”
Unlike traditional VC firms that rely heavily on personal networks, gut feelings, and individual track records, TRAC’s approach systematizes the identification of promising investments. By aggregating the decisions of top-performing VCs and combining this with AI-driven analysis, TRAC eliminates much of the subjectivity inherent in conventional VC decision-making. This data-driven method allows TRAC to cast a wider net and potentially identify opportunities that traditional firms might overlook.
Before launching Fund I, Aaron and Campbell assumed that leveraging the insights of even a single superforecaster would be enough to ensure success, but data proved otherwise. Having one superforecaster investor in a Seed or A Round (first and second deals) is insufficient. However, when two or more such rare birds land at the same spot in the same round, the event becomes highly predictive. In mathematical terms, an order of magnitude higher probability of success.
TRAC’s study of VC superforecasters yielded an unexpected windfall: These super investors can also predict broader market trends with startling accuracy— collectively, not individually.
“We’ve observed a fascinating phenomenon,” Campbell explains. “Two to three months before the economy starts to stall, at the peak of a market, our superforecasters, as a group, step back from making investments. It’s not a coordinated effort—it’s simply that independently, many of them start to see more risk than opportunity.”
The combined behavior of superforecasters appears to serve as an early warning system for economic downturns. With a consistent and repeating pattern, as a group, they provide a level of foresight that traditional economic indicators don’t match.
“Equally intriguing,” Campbell adds, “is that these same superforecasters don’t reenter the market until asset prices have reset to attractive levels. They’re essentially calling the bottom of the market, often before traditional signals suggest a recovery.”
Tapping into this predictive power could be transformative for those with access to the data. Consider an investment firm that could accurately forecast economic cycles, adjusting its strategies months ahead of its competitors. The ability to anticipate both downturns and recoveries could appreciably enhance risk management and profitability.
Without revealing trade secrets, Aaron offers a few tantalizing clues for how TRAC stumbled on the market-predicting abilities of superforecasters. ”The power lies in the aggregate behavior of these exceptional forecasters over time. Individuals are no better at predicting a top or bottom market than you or me,” he says.
The implications of TRAC’s approach extend far beyond venture capital. As AI continues to permeate finance, TRAC’s method may represent the next evolution in predictive technologies, blending the best of human intuition with machine learning capabilities.
However, questions remain about the scalability and long-term reliability of this approach. Critics might argue that the advantage could diminish as more firms adopt similar strategies. Capitalizing on the discovery could also unleash regulatory concerns around data privacy and market manipulation.
Despite these potential hurdles, TRAC’s innovation underscores a crucial insight: in an age of rapid technological advancement, human predictive abilities, when properly harnessed and amplified, can still outperform machines alone.
The frontier of prediction will continue to advance, driven by those who can deftly reduce complex human behavior and insights into ones and zeros. In doing so, we may unlock new realms of predictive power, reaffirming that in the race between humans and machines, the winning formula might just be a collaboration between the two.
Login to add comment
Other posts in this group

Nowadays, when you hear someone talk about faxing, there’s a decent chance it’s the punchline to a groan-inducing dad joke. (Not that I would ever be guilty of such silliness, of course.
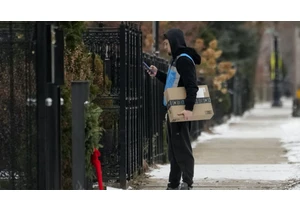
Amazon has agreed to pay nearly $4 million to settle charges that the e-commerce company subsidized its labor costs by taking tips its 


It’s game time for Meta’s wearables: The tech giant has bought two ad spots for its Ray-Ban Meta smart glasses during Sunday’s Super Bowl broadcast, including one that has Chris Hemsworth and Chri

If you scroll through your old photos from the mid-2010s—the golden era of Snapchat—chances are a fai

OpenAI is scouring the U.S. for sites to build a network of huge data centers to power
