When building a full-text search experience such as an FAQ search or Wiki search, there are a number of ways to tackle the challenge using the Elasticsearch Query DSL. For full-text search there’s a relatively long list of possible query types to use, ranging from the simplest match query up to the powerful intervals query. Independent of the query type you choose, you’ll also be faced with understanding and tweaking a list of parameters. While Elasticsearch uses good defaults for query parameters, they can be improved based on the documents in the underlying index (the corpus) and the specific kinds of query strings users will search with. To tackle this task, this post will walk you through the steps and techniques of optimizing a query following a structured and objective process. Before we jump in, let’s consider this example of a multi_match query which searches for a query string on two fields of a document. GET /_search { "query": { "multi_match": { "query": "this is a test", "fields": [ "subject^3", "message" ] } } } Here we’re using the field boost parameter to specify that scores with matches on the subject field should be boosted and multiplied by a factor of three. We do this in an attempt to improve the overall relevance of the query — documents that are the most meaningful with respect to the query should be as close to the top of the results as possible. But how do we choose an appropriate value for the boost? How can we set the boost parameter for not just two fields but a dozen fields? The process of relevance tuning is about understanding the effects of these various parameters. Of all the parameters you could tweak and tune, which ones should you try, with which values, and in which order? While a deep understanding of scoring and relevance tuning shouldn’t be ignored, how can we take a more principled approach to optimizing our queries? Can we use data from user clicks or explicit feedback (e.g., a thumbs up or down on a result) to drive tuning query parameters to improve search relevance? We can, so let’s dive in! To accompany this blog post we’ve put together some example code and Jupyter notebooks that walk you through the steps of optimizing a query with the techniques outlined below. Read this post first, then head over to the code and see all the pieces in action. As of the writing of this post we’re using Elasticsearch 7.10 and everything should work with any of the Elasticsearch licenses. Introducing MS MARCOTo better explain the principles and effects of query parameter tuning, we’re going to be using a public dataset called MS MARCO. The MS MARCO dataset is a large dataset curated by Microsoft Research containing 3.2 million documents scraped from web pages and over 350,000 queries sourced from real Bing web search queries. MS MARCO has a few sub-datasets and associated challenges, so we’re going to focus specifically on the document ranking challenge in this post, as it fits most closely with traditional search experiences. The challenge is effectively to provide the best relevance ranking for a set of selected queries from the MS MARCO dataset. The challenge is open to the public and any researcher or practitioner can participate by submitting their own attempts to come up with the best possible relevance ranking for a set of queries. Later in the post, you’ll see how successful we were by using the techniques outlined here. For the current standings of submissions, you can check out the official leaderboard. Datasets and toolsNow that we have a rough goal in mind of improving relevance by tuning query parameters, let’s have a look at the tools and datasets that we’re going to be using. First let’s outline a more formal description of what we want to achieve and the data we will need.
Given a:
Corpus (documents in an index)
Search query with parameters
Labeled relevance dataset
Metric to measure relevance
Find: Query parameter values that maximize the chosen metric
Labeled relevance datasetRight about now you might be thinking, “wait wait wait, just what exactly is a labeled relevance dataset and where do I get one?!” In short, a labeled relevance dataset is a set of queries with results that have been labeled with a relevance rating. Here’s an example of a very small dataset with just a single query in it: { "id": "query1", "value": "tuning Elasticsearch for relevance", "results": [ { "rank": 1, "id": "doc2", "label_id": 2, "label": "relevant" }, { "rank": 2, "id": "doc1", "label_id": 3, "label": "very relevant" }, { "rank": 3, "id": "doc8", "label_id": 0, "label": "not relevant" }, { "rank": 4, "id": "doc7", "label_id": 1, "label": "related" }, { "rank": 5, "id": "doc3", "label_id": 3, "label": "very relevant" } ] } In this example, we’ve used the relevance labels: (3) very relevant, (2) relevant, (1) related, and (0) not relevant. These labels are arbitrary and you may choose a different scale but the four labels above are pretty common. One way to get these labels is to source them from human judges. A bunch of people can look through your search query logs and for each of the results, provide a label. This can be quite time consuming so many people opt to collect this data from their users directly. They log user clicks and use a click model to convert click activity into relevance labels. The details of this process are well beyond the scope of this blog post but have a look around for presentations and research on click models123. A good place to start is to collect click events for analytics purposes and then look at click models once you have enough behavioural data from users. Have a look at the recent blog post Analyzing online search relevance metrics with Elasticsearch and the Elastic Stack for more. MS MARCO document datasetAs discussed in the introduction, for the purposes of demonstration we’re going to be using the MS MARCO document ranking challenge and associated dataset which has everything we need: a corpus and a labeled relevance dataset. MS MARCO was first built to serve the purpose of benchmarking question and answer (Q&A) systems and all the queries in the dataset are actually questions of some form. For example, you won’t find any queries that look like typical keyword queries like “rules Champions League”. Instead, you will see query strings like “What are the rules of football for the UEFA Champions League?”. Since this is a question answering dataset, the labeled relevance dataset also looks a bit different. Since questions typically have just one best answer, the results have just one “relevant” label (1) and nothing else. Documents are pretty simple and consist of just three fields: url, title, body. Here’s an example (snippet) of a document:
ID: D2286643
URL: http://www.answers.com/Q/Why_is_the_Manhattan_Proj...
Title: Why is the Manhattan Project important?
Body: Answers.com ® Wiki Answers ® Categories History, Politics & Society History War and Military History World War 2
It was the 2nd most secret project of the war (cryptographic work was the 1st). It was the highest priority project of the war, the codeword "silverplate" was assigned to it and overrode all other wartime priorities. It cost $2,000,000,000. Edit Mike M 656 Contributions Answered In US in WW2 Why was the Manhattan project named Manhattan project? The first parts of the Manhattan project took part in the basement of a building located in Manhattan. Edit Pat Shea 3,370 Contributions Answered In War and Military History What is secret project of the Manhattan project? The Manhattan Project was the code name for the WWII project to build the first Nuclear Weapon, the Atomic Bomb. Edit
As you can see, documents have been cleaned and HTML markup has been removed, however they can sometimes contain all sorts of metadata. This is particularly true for user generated content as we see above. Measuring search relevanceOur goal in this blog post is to establish a systematic way to tune query parameters to improve the relevance of our search results. In order to measure how well we are doing with respect to this goal, we need to define a metric that captures how well the results from a given search query satisfy a user’s needs. In other words, we need a way to measure relevance. Luckily we have a tool for this already in Elasticsearch called the Rank Evaluation API. This API allows us to take the datasets outlined above and calculate one of many search relevance metrics. In order to achieve this, the API executes all of the queries from the labeled relevance dataset and compares the results from each query to the labeled results to calculate a relevance metric, such as precision, recall, or mean reciprocal rank (MRR). In our case the MS MARCO document ranking challenge has already selected the mean reciprocal rank (MRR) on the top 100 results (MRR@100) as the relevance metric. This makes sense for a question and answer dataset as MRR only cares about the first relevant document in a result set. It takes the reciprocal rank (1 / rank) of the first relevant document and averages them over all the queries.
Figure 1: MRR formula For the visually inclined, here’s an example calculation of MRR for a small set of queries:
Figure 2: example of an MRR calculation Search templatesNow that we’ve established how we’d like to measure relevance with the help of the Rank Evaluation API, we need to look at how to expose query parameters to allow us to try different values. Recall from our basic multi_match example in the introduction how we set the boost value on the subject field. GET /_search { "query": { "multi_match": { "query": "this is a test", "fields": [ "subject^3", "message" ] } } } When we use the Rank Evaluation API, we specify the metric, the labeled relevance dataset, and optionally the search templates to use for each query. The method we’ll describe below is actually quite powerful since we can rely on search templates. Effectively, we can turn anything that we can parameterize in a search template into a parameter that we can optimize. Here’s another multi_match query but using the real fields from the MS
Connectez-vous pour ajouter un commentaire
Autres messages de ce groupe
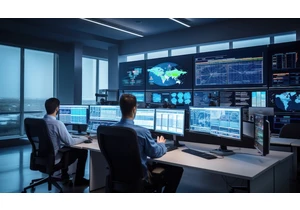
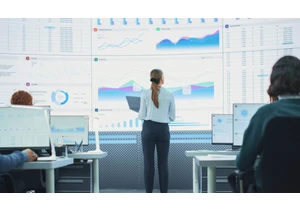
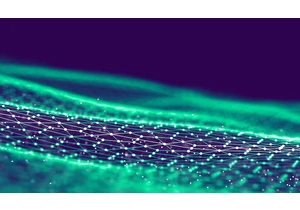
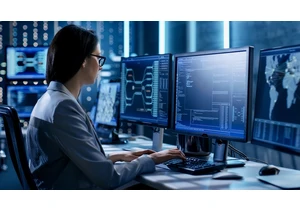
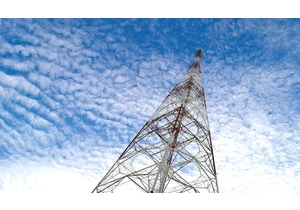
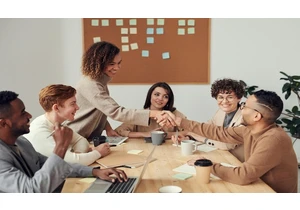