Everything is more difficult in space, and learning software is no exception. But the pressure to push AI into everything can’t be denied.
U.S. satellite operator Spire will work with Mission Control, a Canadian space software company, on a demo mission to launch in 2025 that will see a Spire-built Earth observation (EO) satellite use Mission Control machine learning models to perform analyses in orbit.
Founded in 2015, Mission Control develops software for autonomous space operations. The company was among the first to deploy a deep-learning algorithm in Earth orbit, and the first to do so in lunar orbit during Ispace’s first attempt to land on the moon.
We caught up with Mission Control CTO Michele Faragalli to understand what the mission is set to prove.
This interview has been condensed and edited for clarity.
How does Mission Control fit into the world of AI development?
There [are] a lot of companies out there, especially terrestrial tech companies, that are really leading the way in terms of those [deep learning] models. But we have our own subset of challenges in space—you might have lower power avionics, and so you really have to look at tiny models. Using terrestrial software infrastructure and terrestrial computing technology is a very different type of problem than what we’re doing for very low-power processors that have to fit on a lunar micro rover or a CubeSat.
Why do spacecraft need advanced machine learning models?
The latency is so high, the bandwidth restrictions are so limited, and, frankly, costs are so prohibitive that you just need the spacecraft to do more on its own. Let’s say you’re monitoring a flood or wildfires. The speed of response could be enabled by your satellite processing the data collected immediately on board, rather than waiting for your satellite to pass and then downlinking that.
What makes using AI models in space such a challenge?
What we learned through these demonstration missions very quickly was that you can train these models before flight, but the reality is once you have them up in orbit or on the surface of the moon, the operating conditions are just completely different. . . . How do you train a computer vision model that needs to detect life on Mars, as a random example? You have to build synthetic data, or you have to go to analog sites on Earth. The reality is, it’s never going to be perfect.
How do you try to solve that problem?
A big genesis for this project we’ve just announced with Spire is looking at, how do you handle the uncertainty of spaceflight? In terrestrial edge AI sectors, you would call on an MLOPs pipeline, a machine learning operations pipeline. How do you retrain those models using new data? How do you deploy them to the hardware, to the edge device, or, in this case, to the spacecraft, with an over-the-air software update, and then monitor that performance so you’re ensuring you’re maintaining that persistent performance of that asset?
What else makes it challenging to deploy AI on space missions?
There’s just really no certification standard yet for this type of flight software. As an example, a space agency doing a Class A mission would likely not impose a requirement on using deep learning techniques, because there may or may not be a way in which they could validate that.
These are statistical approaches, right? They have a certain kind of statistical performance output, 90% accuracy. So say you have a 90% accuracy, one of the challenges is you don’t know when it won’t perform. It’s not a physics-based problem that you can validate when it’s going to fail, and that’s a challenge for trusting these algorithms to perform under any operating conditions.
<hr class=“wp-block-separator is-style-wide”/>
This story originally appeared on Payload and is republished here with permission.
Connectez-vous pour ajouter un commentaire
Autres messages de ce groupe

Is LinkedIn the new TikTok?
Short-form video is now the fastest-growing category on LinkedIn, growing at twice the rate of other post formats on the platform. According to LinkedIn

Robinhood said on Tuesday it is rolling back the event contracts that would let users bet on the result of the
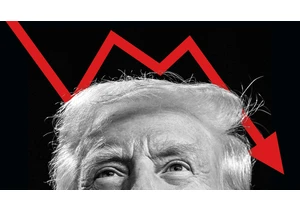
Donald Trump drew plenty of criticism by launching his own branded memecoin three days before his

The AI search firm Perplexity routinely lets users try out state-of-the-art large language models on its site, but the company moved quickly to put Chinese company DeepSeek’s new R1 model front an

Nintendo’s profits tumbled as sales of its Switch console lost momentum, prompting the

“We want grandparents who want to have pizza nights with us, attend baseball and basketball games, have ice cream dates, take bike rides, just genuinely have fun with us and our boys,” reads one p

Apple rolled out its newest iPhone app called Invites, which lets iCloud+ subs