Welcome back! In the last blog we set up bidirectional communication between ServiceNow and Elasticsearch. We spent most of our time in ServiceNow, but from here on, we will be working in Elasticsearch and Kibana. By the end of this post, you'll have these two powerful applications working together to make incident management a breeze. Or at least a lot easier than you may be used to!
As with all Elasticsearch projects, we will create indices with mappings that suit our needs. For this project, we need indices that can hold the following data:
- ServiceNow incident updates: This stores all information coming from ServiceNow to Elasticsearch. This is the index that ServiceNow pushes updates to.
- Application uptime summary for ease of use: This is going to store how many total hours each application has been online. Consider this an intermediate data state for ease of use.
- Application incident summary: This is going to store how many incidents each application has had, each application’s uptime, and the current MTBF (mean time between failure) for each application.
The last two indices are helper indices so that we don’t have to have a whole bunch of complicated logic running every time we refresh the Canvas workpad we'll create in part 3. They are going to be updated continuously for us through the use of transforms.
Create the three indices
To create the indices, you'll use the below guidance. Note that if you don't use the same names as used below, you might need to make adjustments in your ServiceNow setup.
servicenow-incident-updates
Following best practices, we are going to set up an index template, index alias, and then an index lifecycle management (ILM) policy. We will also create an index template so that the same mapping is applied to any future index created by our ILM policy. Our ILM policy is going to create a new index once 50GB of data is stored within the index and then delete it after 1 year. An index alias is going to be used so we can easily point towards the new index when it’s created without updating our ServiceNow business rule.
# Create the ILM policy PUT _ilm/policy/servicenow-incident-updates-policy { "policy": { "phases": { "hot": { "actions": { "rollover": { "max_size": "50GB" } } }, "delete": { "min_age": "360d", "actions": { "delete": {} } } } } }
# Create the index template PUT _template/servicenow-incident-updates-template { "index_patterns": [ "servicenow-incident-updates*" ], "settings": { "number_of_shards": 1, "index.lifecycle.name": "servicenow-incident-updates-policy", "index.lifecycle.rollover_alias": "servicenow-incident-updates" }, "mappings": { "properties": { "@timestamp": { "type": "date", "format": "yyyy-MM-dd HH:mm:ss" }, "assignedTo": { "type": "keyword" }, "description": { "type": "text", "fields": { "keyword": { "type": "keyword", "ignore_above": 256 } } }, "incidentID": { "type": "keyword" }, "state": { "type": "keyword" }, "app_name": { "type": "keyword" }, "updatedDate": { "type": "date", "format": "yyyy-MM-dd HH:mm:ss" }, "workNotes": { "type": "text" } } } }
# Bootstrap the initial index and create the alias PUT servicenow-incident-updates-000001 { "aliases": { "servicenow-incident-updates": { "is_write_index": true } } }
app_uptime_summary & app_incident_summary
As both of these indices are entity-centric, they do not need to have an ILM policy associated with them. This is because we will only ever have one document per application that we are monitoring. To create the indices, you issue the following commands:
PUT app_uptime_summary { "mappings": { "properties": { "hours_online": { "type": "float" }, "app_name": { "type": "keyword" }, "up_count": { "type": "long" }, "last_updated": { "type": "date" } } } }
PUT app_incident_summary { "mappings": { "properties" : { "hours_online" : { "type" : "float" }, "incident_count" : { "type" : "integer" }, "app_name" : { "type" : "keyword" }, "mtbf" : { "type" : "float" } } } }
Set up the two transforms
Transforms are an incredibly useful and recent addition to the Elastic Stack. They provide the capability to convert existing indices into an entity-centric summary, which is great for analytics and new insights. An often overlooked benefit of transforms is their performance benefits. For example, instead of trying to calculate the MTBF for each application by query and aggregation (which would get quite complicated), we can have a continuous transform calculating it for us on a cadence of our choice. For example, every minute! Without transforms they would be calculated once for every refresh that each person does on the Canvas workpad. Meaning, if we have 50 people using the workpad with a refresh interval of 30 seconds, we run the expensive query 100 times per minute (which seems a bit excessive). While this wouldn’t be an issue for Elasticsearch in most cases, I want to take advantage of this awesome new feature which makes life much easier.
We are going to create two transforms:
- calculate_uptime_hours_online_transform: Calculates the number of hours each application has been online and responsive. It does this by utilizing the uptime data from Heartbeat. It will store these results in the
app_uptime_summary
index.
- app_incident_summary_transform: combines the ServiceNow data with the uptime data coming from the previously mentioned transform (yes... sounds a bit like a join to me). This transform will take the uptime data and work out how many incidents each application has had, bring forward how many hours it has been online and finally calculate the MTBF based on those two metrics. The resulting index will be called
app_incident_summary
.
calculate_uptime_hours_online_transform
PUT _transform/calculate_uptime_hours_online_transform { "source": { "index": [ "heartbeat*" ], "query": { "bool": { "must": [ { "match_phrase": { "monitor.status": "up" } } ] } } }, "dest": { "index": "app_uptime_summary" }, "sync": { "time": { "field": "@timestamp", "delay": "60s" } }, "pivot": { "group_by": { "app_name": { "terms": { "field": "monitor.name" } } }, "aggregations": { "@timestamp": { "max": { "field": "@timestamp" } }, "up_count": { "value_count": { "field": "monitor.status" } }, "hours_online": { "bucket_script": { "buckets_path": { "up_count": "up_count" }, "script": "(params.up_count * 60.0) / 3600.0" } } } }, "description": "Calculate the hours online for each thing monitored by uptime" }
app_incident_summary_transform
PUT _transform/app_incident_summary_transform { "source": { "index": [ "app_uptime_summary", "servicenow*" ] }, "pivot": { "group_by": { "app_name": { "terms": { "field": "app_name" } } }, "aggregations": { "incident_count": { "cardinality": { "field": "incidentID" } }, "hours_online": { "max": { "field": "hours_online", "missing": 0 } }, "mtbf": { "bucket_script": { "buckets_path": { "hours_online": "hours_online", "incident_count": "incident_count" }, "script": "(float)params.hours_online / (float)params.incident_count" } } } }, "description": "Calculates the MTBF for apps by using the output from the calculate_uptime_hours_online transform", "dest": { "index": "app_incident_summary" }, "sync": { "time": { "field": "@timestamp", "delay": "1m" } } }
Let’s now ensure that both transforms are running:
POST _transform/calculate_uptime_hours_online_transform/_start POST _transform/app_incident_summary_transform/_start
Uptime alerts to create tickets in ServiceNow
Accedi per aggiungere un commento
Altri post in questo gruppo
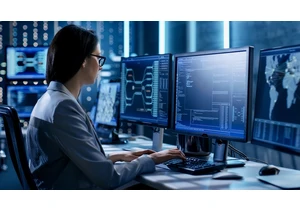
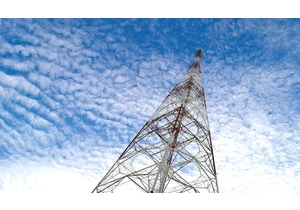
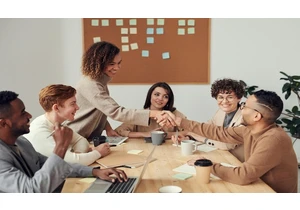
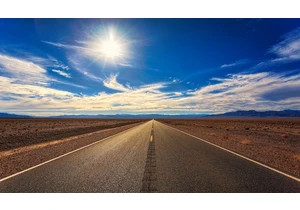
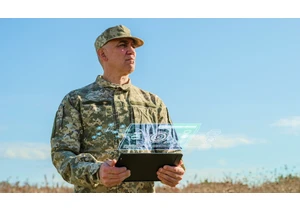
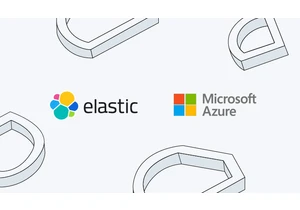
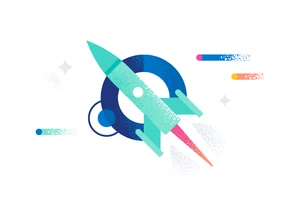
Version 7.17.29 of the Elastic Stack was released today. We recommend you upgrade to this latest version. We recommend 7.17.29 over the previous versi