Full-text search is a common — but very important — part of great search experience. However, full-text search can be difficult to implement in some languages, which is the case with Japanese. In this blog, we’ll explore the challenges of implementing full-text search in Japanese and demonstrate some ways you can overcome these challenges in Elasticsearch. So, what is full-text search?Per wiki, here is the formal definition: In text retrieval, full-text search refers to techniques for searching a single computer-stored document or a collection in a full-text database. Full-text search is distinguished from searches based on metadata or on parts of the original texts represented in databases (such as titles, abstracts, selected sections, or bibliographical references). In non-technical terms, full-text search is what powers a lot of the digital experiences you have today. It's the type of search that will try to find a word or phrase anywhere it could be hiding in a dataset. So when you are shopping online and search for "phone," full-text search would bring back any product that: has been classified as a phone, has phone in the description, has phone in the manufacturer's name, etc. This includes matches on "telephone," "saxophone," etc. This type of search is extremely convenient for finding what you want as quickly as possible. It's also extremely inconvenient if the search engine isn't properly configured and managed. When you search for "phone," you're probably not searching for a saxophone, and a well-maintained search engine knows that. And this is where things get interesting! Precision and recallRecall and precision are the two common ways to measure the quality of a full-text search system. Precision represents "how small the search omissions are" and recall represents "how small the search noise is." For example, a precise search may only return results for "phone" when an item is classified as a phone. A search with high recall would return all items that have the word "phone" in it, even saxophones. There is always a trade-off between precision and recall, so you need to decide for yourself what's best for your use case, then test and adjust it incrementally. Inverted index and analyzeYou are familiar with the index in the back of a book. The useful terms from the book are sorted and listed together with page numbers so you know where to find that term. The similar inverted index is used in Elasticsearch for full-text search.
At index time, text strings are analyzed and added to the inverted index making them very easy to find. Also, the query string at search time is analyzed too. Different filters (e.g., lowercase, stop words, stemming, etc.) could be applied in analyzing process flow. Using the same analyzer for both search and indexing is a common use case. And for special use cases such as the Japanese full-text search we will talk about in this blog, different ones could be applied too. This string analysis process creates a detailed index of words and phrases, making the data full-text searchable. What’s different about full-text search in Japanese?That's a good question. Before I answer it, let me ask it again… 日本語での全文検索は英語と何が違いますか? That's the same question, but it looks very different. And this is why full-text search is different too. Word breaks don’t depend on whitespaceTo analyze a text string, an analyzer is required. In most European languages (including English), words are separated with whitespace, which makes it easy to divide a sentence into words. However, in Japanese, individual words are not separated with whitespace. So how do we do it? Two methods to analyze Japanese wordsSince Japanese does not recognize word breaks on whitespace, the inverted index is mainly created by the following two methods.
n-gram analysis: Separate text strings by N characters
Morphological analysis: Divide into meaningful words using a dictionary
However, each of these on their own is not enough:
In n-gram, the index tends to be bloated. Processing based on part-of-speech information is impossible and there are many meaningless divisions. (Fewer search omissions, but more search noise.)
Morphological analysis is weak with new words (unknown words). In a dictionary-based case, words that are not in the dictionary cannot be detected. (Less search noise but more search omissions.)
As you can see, this is exactly the above-mentioned trade-off. Japanese full-text search needs both!Based on the above analysis gaps, Japanese full-text search should use both analysis types, with the strengths of each making up for their different weaknesses.
Fewer search omissions when using n-gram analysis
Less search noise when using morphological analysis
With these analyses working together, full-text search is possible. Implementing Japanese full-text search using both morphological and n-gram analysis with ElasticsearchSuch situations can be handled by using Elasticsearch, thus making it possible to implement Japanese full-text search that emphasizes both precision and recall. Implementation summary and exampleIn short, you will need to define two types of analyzers for n-gram and morphological analysis in your index mappings and settings, and then assign them to each field. At index time, you'll create the inverted indices via these two types of analyzers. At search time, you'll search both of the two inverted indices. Before going into the detailed explanation, let's first look at an example of implementing full-text search in Japanese. Example implementation requirements Japanese full-text search is possible. For example, if you search for "東京大学" (University of Tokyo), documents including "東京大学" will be displayed. Also, if you search for "米国," (US) documents that include "米国" will be displayed. In addition, users can search for synonyms. For example, if you search for "東京大学," documents including "東大" (short way to say "東京大学") will be displayed. Also, if you search for "米国," documents that include "アメリカ" (America) will be displayed. Preparation for example implementation Install the analysis-icu and analysis-kuromoji plugins for analysis. Create an index for full-text search. In this example, the index name is my_full text_search. Then use my_field as the field for full-text search. Example: Configuration of index settings and mappingsPUT my_full text_search { "settings": { "analysis": { "char_filter": { "normalize": { "type": "icu_normalizer", "name": "nfkc", "mode": "compose" } }, "tokenizer": { "ja_kuromoji_tokenizer": { "mode": "search", "type": "kuromoji_tokenizer", "discard_compound_token": true, "user_dictionary_rules": [ "東京スカイツリー,東京 スカイツリー,トウキョウ スカイツリー,カスタム名詞" ] }, "ja_ngram_tokenizer": { "type": "ngram", "min_gram": 2, "max_gram": 2, "token_chars": [ "letter", "digit" ] } }, "filter": { "ja_index_synonym": { "type": "synonym", "lenient": false, "synonyms": [ ] }, "ja_search_synonym": { "type": "synonym_graph", "lenient": false, "synonyms": [ "米国, アメリカ", "東京大学, 東大" ] } }, "analyzer": { "ja_kuromoji_index_analyzer": { "type": "custom", "char_filter": [ "normalize" ], "tokenizer": "ja_kuromoji_tokenizer", "filter": [ "kuromoji_baseform", "kuromoji_part_of_speech", "ja_index_synonym", "cjk_width", "ja_stop", "kuromoji_stemmer", "lowercase" ] }, "ja_kuromoji_search_analyzer": { "type": "custom", "char_filter": [ "normalize" ], "tokenizer": "ja_kuromoji_tokenizer", "filter": [ "kuromoji_baseform", "kuromoji_part_of_speech", "ja_search_synonym", "cjk_width", "ja_stop", "kuromoji_stemmer", "lowercase" ] }, "ja_ngram_index_analyzer": { "type": "custom", "char_filter": [ "normalize" ], "tokenizer": "ja_ngram_tokenizer", "filter": [ "lowercase" ] }, "ja_ngram_search_analyzer": { "type": "custom", "char_filter": [ "normalize" ], "tokenizer": "ja_ngram_tokenizer", "filter": [ "ja_search_synonym", "lowercase" ] } } } }, "mappings": { "properties": { "my_field": { "type": "text", "search_analyzer": "ja_kuromoji_search_analyzer", "analyzer": "ja_kuromoji_index_analyzer", "fields": { "ngram": { "type": "text", "search_analyzer": "ja_ngram_search_analyzer", "analyzer": "ja_ngram_index_analyzer" } } } } } } Example: Data preparationAs mentioned in the above requirements, prepare documents that include the words "米国" (US), "アメリカ" (America), "東京大学" (University of Tokyo), and "東大" (short way to say "東京大学).
Data preparation
POST _bulk {"index": {"_index": "my_full text_search", "_id": 1}} {"my_field": "アメリカ"} {"index": {"_index": "my_full text_search", "_id": 2}} {"my_field": "米国"} {"index": {"_index": "my_full text_search", "_id": 3}} {"my_field": "アメリカの大学"} {"index": {"_index": "my_full text_search", "_id": 4}} {"my_field": "東京大学"} {"index": {"_index": "my_full text_search", "_id": 5}} {"my_field": "帝京大学"} {"index": {"_index": "my_full text_search", "_id": 6}} {"my_field": "東京で夢の大学生活"} {"index": {"_index": "my_full text_search", "_id": 7}} {"my_field": "東京大学で夢の生活"} {"index": {"_index": "my_full text_search",
Autentifică-te pentru a adăuga comentarii
Alte posturi din acest grup
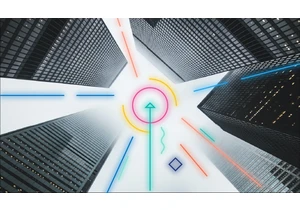
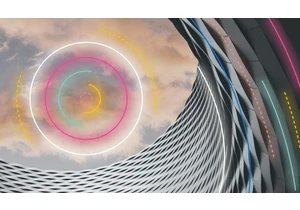
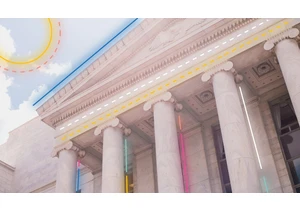
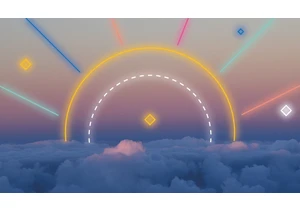
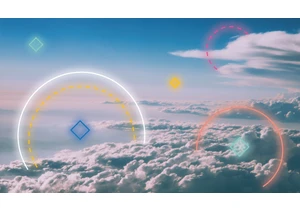
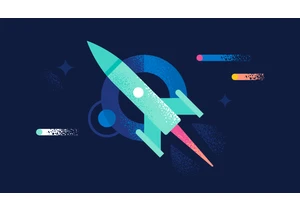
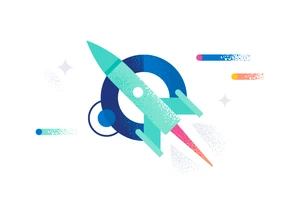