Recently, the JetBrains .NET advocacy team published a deep-dive post powered by data we retrieved from the official NuGet APIs with the goal of better understanding our community's OSS past and trying to predict trends into the future. This resulted in a giant dataset. Given our experience with Elasticsearch, we knew that the best tool to process millions of records was what we're calling the NECK stack: .NET, Elasticsearch, CSV, and Kibana. In this blog, we'll explore what it took to retrieve the millions of package records, process them using .NET and JetBrains Rider, index them into Elasticsearch via the NEST client, and ultimately build the Kibana dashboards we used to generate our reports. The NuGet API and DataMost technology stacks have adopted open source and dependency management as core tenets, and Microsoft and .NET have done that enthusiastically so. For those unfamiliar with the .NET ecosystem, NuGet is the official package management protocol and service for .NET developers. The NuGet ecosystem has grown substantially since its initial release in 2011, starting with a handful of packages to today's service hosting over 231,181 unique packages and close to 3 million permutations; that's a lot of data. Luckily, Maarten Balliauw has done much of the heavy lifting to understand and retrieve the data from the NuGet API. In summary, we were able to loop through the NuGet API and retrieve the following pieces of information: Authors, icon URL, package Id, listing status, project URL, publish date, tags, target frameworks, package URL, package version, download numbers, and other unimportant data. Once the process was complete, we had generated a 1.5 GB CSV file during our retrieval of data, likely the most massive CSV file we've ever seen. We attempted to open this file in some commonly-used spreadsheet tools like Excel, Google Spreadsheets, and Apple Numbers with no success, and frankly didn't have much hope of it working. Here's a small sample of that data. PartitionKey,RowKey,Timestamp,Authors:String,IconUrl:String,Id:String,IsListed:Boolean,LicenseUrl:String,ProjectUrl:String,Published:DateTime,Tags:String,TargetFrameworks:String,Url:String,Version:String,VersionNormalized:String,VersionVerbatim:String,DownloadCount:Long,DownloadCountForAllVersions:Long,PackageType:String,IsVerified:Boolean 03.ADSFramework.Logging,1.0.0,2020-10-30T06:49:21.0291480Z,"ADSBI, Inc.",https://github.com/nathanadsbi/ADSIcon/blob/master/ads.ico?raw=true,03.ADSFramework.Logging,False,,"",1900-01-01T00:00:00.0000000Z,03.ADSBI 03.ADSFramework.Logging,"[""net461""]",https://globalcdn.nuget.org/packages/03.adsframework.logging.1.0.0.nupkg,1.0.0,1.0.0,1.0.0,,,, 03.ADSFramework.Logging,1.0.2,2020-10-30T06:49:22.4903642Z,"ADSBI, Inc.",https://github.com/nathanadsbi/ADSIcon/blob/master/ads.ico?raw=true,03.ADSFramework.Logging,False,,"",1900-01-01T00:00:00.0000000Z,03.ADSBI 03.ADSFramework.Logging,"[""net461""]",https://globalcdn.nuget.org/packages/03.adsframework.logging.1.0.2.nupkg,1.0.2,1.0.2,1.0.2,,,, 03.ADSFramework,1.0.0,2020-10-30T05:29:51.6321787Z,"Nathan Sawyer, Patrick Della Rocca, Shannon Fisher","",03.ADSFramework,False,,"",1900-01-01T00:00:00.0000000Z,"","[""net461"",""netstandard2.0""]",https://globalcdn.nuget.org/packages/03.adsframework.1.0.0.nupkg,1.0.0,1.0.0,1.0.0,,,,We chose to represent the data in a comma-delimited format to allow for easy consumption of the information, which we'll see in the next section. .NET Console ProcessingSince adopting a cross-platform mantra, .NET has been a lot more interesting from a tooling and data-processing perspective. Developers can now write and execute the same code across all major operating systems: Windows, Linux, and macOS. As JetBrains .NET advocates, we love C#, and we also love the Elasticsearch client library, NEST, developed and maintained by Elastic. We were also able to tap into the OSS ecosystem and utilize the fantastic CsvHelper library, which makes processing CSV files effortless. Let's take a look at how we harnessed the OSS .NET ecosystem's power to consume and load 1.5 GB of data into Elasticsearch. Processing CSVs using CSVHelperCSV files aren't incredibly difficult to process, primarily when CsvHelper contributors have handled much of the hard work of determining and solving edge cases. To get started, we first need to install the NuGet package into our Console application, along with Newtonsoft.Json, a library designed to work with JSON.
Once we install the package, we'll need to create a ClassMap definition. A ClassMap allows us to define which corresponding CSV columns we assign to our C# class properties. Like most data projects, our data is rarely perfect, and we need to account for strange edge cases and broken rows. We can also take this opportunity to normalize data before it goes into our Elasticsearch index. public class NugetRecordMap : ClassMap { public NugetRecordMap() { string [] ToStringArray(string value) { if (string.IsNullOrWhiteSpace(value)) return new string [0];
try
{
// just because we have brackets doesn't mean
// we have a JSON Array... trust me
if (
value.StartsWith("[") &&
value.EndsWith("]") &&
value.Count(x => x == '[') == 1 &&
value.Count(x => x == ']') == 1)
{
return DeserializeObject(value);
}
}
catch
{
}
try
{
return value
.Replace("[", string.Empty)
.Replace("]", string.Empty)
.Split(' ', StringSplitOptions.TrimEntries | StringSplitOptions.RemoveEmptyEntries);
}
catch
{
}
return new string[0];
}
var exclude = new [] { "LLC", "Inc." };
// used for Elasticsearch
Map(m => m.Id).Ignore();
Map(m => m.License).Ignore();
Map(m => m.PartitionKey).Name("PartitionKey");
Map(m => m.RowKey).Name("RowKey");
Map(m => m.Authors).ConvertUsing(r =>
{
return r
.GetField("Authors:String")?
.ToLowerInvariant()
.Replace("and other contributors", string.Empty)
.Replace("and contributors", string.Empty)
.Split(',', StringSplitOptions.RemoveEmptyEntries | StringSplitOptions.TrimEntries)
.Except(exclude, StringComparer.OrdinalIgnoreCase)
.ToArray();
}
);
Map(m => m.IconUrl).Name("IconUrl:String");
Map(m => m.PackageId).Name("Id:String");
Map(m => m.IsListed).Name("IsListed:Boolean");
Map(m => m.LicenseUrl).Name("LicenseUrl:String");
Map(m => m.ProjectUrl).Name("ProjectUrl:String");
Map(m => m.Published).Name("Published:DateTime");
Map(m => m.Tags).ConvertUsing(r => ToStringArray(r.GetField("Tags:String")).Select(x => x.ToLowerInvariant()).ToArray());
Map(m => m.TargetFrameworks).ConvertUsing(r => ToStringArray(r.GetField("TargetFrameworks:String")));
Map(m => m.Url).Name("Url:String");
Map(m => m.Version).Name("Version:String");
Map(m => m.VersionNormalized).Name("VersionNormalized:String");
Map(m => m.VersionVerbatim).Name("VersionVerbatim:String");
Map(m => m.Prefix).ConvertUsing(r => {
var id = r.GetField("Id:String");
if (id.Contains('.')) {
return id.Substring(0, id.IndexOf('.'));
}
return id.ToLowerInvariant();
});
Map(m => m.DownloadCount).ConvertUsing(m => {
var field = m.GetField("DownloadCount:Long");
if (long.TryParse(field, out var value))
return value;
return null;
});
Map(m => m.DownloadCountForAllVersions).ConvertUsing(m => {
var field = m.GetField("DownloadCountForAllVersions:Long");
if (long.TryParse(field, out var value))
return value;
return null;
});
Map(m => m.PackageType).ConvertUsing(m => {
var field = m.GetField("PackageType:String");
return string.IsNullOrWhiteSpace(field) ? "Dependency" : field;
});
Map(m => m.IsVerified).ConvertUsing(m => {
var field = m.GetField("IsVerified:Boolean");
if (bool.TryParse(field, out var value))
return value;
return false;
});
}
}A good general rule when working with Elasticsearch is to clean as much of the data before indexing. Folks may have noticed that in the example rows, some of the columns contained arrays. Handling non-flat data in a flat representation means we need to take approaches to maintain data integrity without compromising on the simple format. In our case, we chose array syntax as we know Elasticsearch can straightforwardly handle array fields. Eagle-eyed C# developers may have also recognized the empty catch blocks. We found a few lines in the 2.7 million rows that we could not process in our application runs. We erred on the side of processing the most records we could, rather than all of them. In the end, five rows were incorrect due to syntax issues. Folks considering this approach should consider error handling and whether data loss is acceptable for their use case. Defining Our Index With NESTLike CSVHelper, we can retrieve the NEST package from NuGet. NuGet package versions for NEST should match the version of our Elasticsearch instance. In this case, we are using Elasticsearch 7.9.0, but there are no specific features that we are utilizing that are exclusive to this particular version.
Next, we need to define our Elasticsearch index. Kibana will use our index to allow us to run interesting queries and generate meaningful dashboards. Luckily, NEST enables us to define indexes using C# objects and attributes. ElasticsearchType(IdProperty = "Id", RelationName = "packag
Autentifică-te pentru a adăuga comentarii
Alte posturi din acest grup
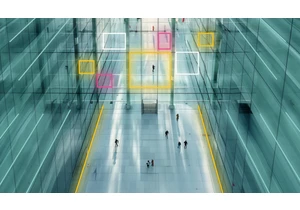
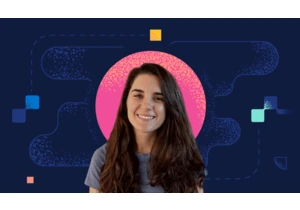
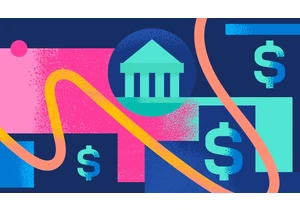
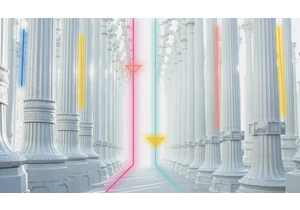
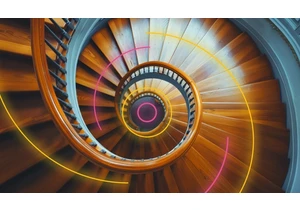
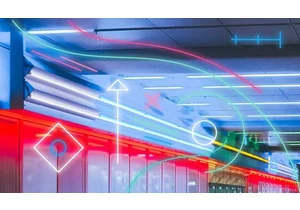