Have you ever tried to search for objects in images? Elasticsearch can help you store, analyze, and search for objects in images or videos. In this quick tutorial, we’ll show you how to build a system for facial recognition with Python. Learn more about how to detect and encode facial information — and find matches in search. A quick walkthrough of the basicsNeed a refresher? Let's briefly review some fundamentals about a few basic concepts. Facial recognitionFacial recognition is the process of identifying a person using their face — for example, for the purpose of implementing an authentication mechanism (like unlocking your smartphone). It captures, analyzes, and compares patterns based on the person's facial details. This process can be divided into three phases:
Face detection: Identify human faces in digital images
Face data encoding: Convert facial features into digital representation
Face match: Search and compare facial features
We’ll walk you through each phase in our example. 128-dimension vectorFacial features can be transformed into a set of digital information in order to be stored and analysed.
Create a getFaces.py file: touch getVectorFromPicture.py Append the file with following script: import face_recognition import numpy as np import sys image = face_recognition.load_image_file("$PATH_TO_IMAGE")
detect the faces from the images
face_locations = face_recognition.face_locations(image)
encode the 128-dimension face encoding for each face in the image
face_encodings = face_recognition.face_encodings(image, face_locations)
Display the 128-dimension for each face detected
for face_encoding in face_encodings: print("Face found ==> ", face_encoding.tolist()) Let’s execute getVectorFromPicture.py to get the facial features representation for images of Elastic’s founders. The $PATH_TO_IMAGE variable needs to be changed in the script to set the image file name.
We can now store the facial features representation into Elasticsearch.
Let’s first create the index with mapping that contains a field with dense_vector type:
Store the face 128-dimension in Elasticsearch
Create the mapping
curl -XPUT "http://localhost:9200/faces" -H 'Content-Type: application/json' -d' { "mappings" : { "properties" : { "face_name" : { "type" : "keyword" }, "face_encoding" : { "type" : "dense_vector", "dims" : 128 } } } }' We need to create one document for each face representation, which can be done using the Index API:
Index the face feature representation
curl -XPOST "http://localhost:9200/faces/_doc" -H 'Content-Type: application/json' -d' { "face_name": "name", "face_encoding": [ -0.14664565, 0.07806452, 0.03944433, ... ... ... -0.03167224, -0.13942884 ] }'
Match the facesLet's say we have indexed four documents in Elasticsearch that contain each facial representation of Elastic’s founders. We can now use another image of our founders to match the individual images.
Create a recognizeFaces.py file: touch recognizeFaces.py In this script, we’ll extract the vectors for each face detected from the input image, and we use the vectors for each face to build the query to send to Elasticsearch: Import the libraries: import face_recognition import numpy as np from elasticsearch import Elasticsearch import sys Add the following section to connect to Elasticsearch:
Connect to Elasticsearch cluster
from elasticsearch import Elasticsearch es = Elasticsearch( cloud_id="cluster-1:dXMa5Fx...", http_auth=("elastic", ""), ) We will use the cosineSimilarity function to calculate the measure of cosine similarity between a given query vectors and document vectors stored into Elasticsearch. i=0 for face_encoding in face_encodings: i += 1 print("Face",i) response = es.search( index="faces", body={ "size": 1, "_source": "face_name", "query": { "script_score": { "query" : { "match_all": {} }, "script": { "source": "cosineSimilarity(params.query_vector, 'face_encoding')", "params": { "query_vector":face_encoding.tolist() } } } } } ) Let’s assume the score under 0.93 is considered an unknown face: for hit in response['hits']['hits']:
double score=float(hit['_score'])
if (float(hit['_score']) > 0.93):
print("==> This face match with ", hit['_source']['my_text'], ",the score is" ,hit['_score'])
else:
print("==> Unknown face")
Let's execute our script:
The script was able to detect all faces with score match higher than 0.93. Go a step further with advanced searchFacial recognition and search can be combined for advanced use cases. You can use Elasticsearch to build more sophisticated queries like geo-queries, query-dsl-bool-query, and search-aggregations. As an example, the following query applies cosineSimilarity search on a specific location within a 200-km radius: GET /_search { "query": { "script_score": { "query": { "bool": { "must": { "match_all": {} }, "filter": { "geo_distance": { "distance": "200km", "pin.location": { "lat": 40, "lon": -70 } } } } }, "script": { "source": "cosineSimilarity(params.query_vector, 'face_encoding')", "params": { "query_vector":[ -0.14664565, 0.07806452, 0.03944433, ... ... ... -0.03167224, -0.13942884 ] } } } } } Combining cosineSimilarity with other Elasticsearch queries give you unlimited possibilities to implement more complex use cases. ConclusionFacial recognition can be relevant in many use cases, and you may already use it in your daily life. The concepts described above can be generalized to any object detection in images or videos, so you may extend your use case to a very large utilization. Elasticsearch can help simplify your advanced requirements. Try it out with a free 14-day trial of Elastic Cloud, our official managed Elasticsearch offering, and let us know what you think in our Discuss forums.
https://www.elastic.co/blog/how-to-build-a-facial-recognition-system-using-elasticsearch-and-python
Autentifică-te pentru a adăuga comentarii
Alte posturi din acest grup
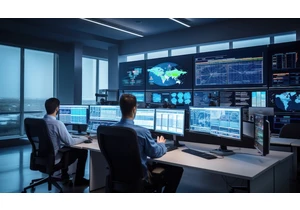
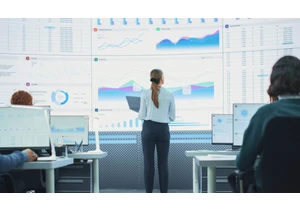
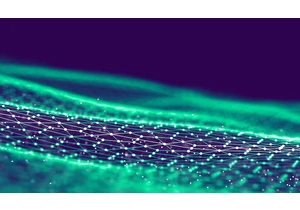
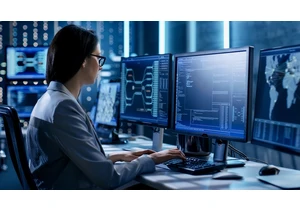
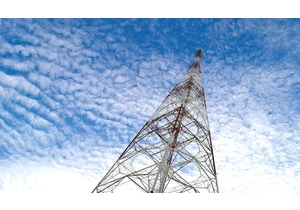
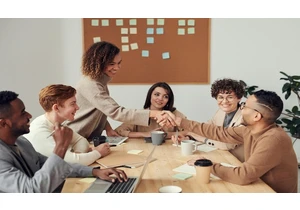