Why are AI chatbots so intelligent—capable of understanding complex ideas, crafting surprisingly good short stories, and intuitively grasping what users mean? The truth is, we don’t fully know. Large language models “think” in ways that don’t look very human. Their outputs are formed from billions of mathematical signals bouncing through layers of neural networks powered by computers of unprecedented power and speed, and most of that activity remains invisible or inscrutable to AI researchers.
This opacity presents obvious challenges, since the best way to control something is to understand how it works. Scientists had a firm grasp of nuclear physics before the first bomb or power plant was built. The same can’t be said for generative AI models. Researchers working in the AI safety subfield of “mechanistic interpretability” who spend their days studying the complex sequences of mathematical functions that lead to an LLM outputting its next word or pixel, are still playing catch-up. The good news is that they’re making real progress. Case in point: the release of a pair of new research papers from Anthropic that contain fresh insights into LLMs’ internal “thinking.”
Just as the parameters inside neural networks are based on “neurons” in the brain, the Anthropic researchers looked to neuroscience for ways of studying AI. Anthropic research scientist Joshua Batson tells Fast Company that his team developed a research tool—a sort of “AI microscope”—that can follow the data patterns and information flows within an LLM, observing how it links words and concepts en route to an answer. A year ago, the researchers could see only specific features of these patterns and flows, but they’ve now begun to observe how one idea leads to another through a sequence of reasoning.
“We’re trying to connect that all together and basically walk through step-by-step when you put a prompt into a model why it says the next word,” Batson says. “And since the model’s [answers] happen one word at a time, if you can break it down and just say, ‘Well, why did it say this word instead of that word?’ then you can kind of unpack the whole thing.”
AI thinks differently—even when it comes to simple math
The research reinforces the idea that AI systems approach problems very differently than human beings do. LLMs aren’t explicitly taught tasks like arithmetic. Rather, they’re shown correct answers and left to develop their own probabilistic path toward that conclusion. Batson and his team studied a simple example of this math—asking an 18-layer test LLM to add the numbers 36 and 59—and found the AI’s “process” was very different from the average human’s calculation.
Rather than performing a human-like step-by-step, the test model used two kinds of logic to arrive at the answer: It approximated the answer (is it in the 90s?) and it estimated the last digit of the answer. By combining the probabilities of various answers, Claude was able to arrive at the correct sum. “It definitely learned a different strategy for doing the math than the one that you or I were taught in school,” Batson says.
Thinking in universal concepts
The researchers also studied whether LLMs, which often analyze and generate content in many languages, necessarily “think” in the language of the words given to it in the user’s prompt. “Is it using just English [words] when it’s doing English stuff and French parts when it’s doing French stuff and Chinese parts when it’s doing Chinese stuff?” Batson asks. “Or are there some parts of the model that are actually thinking in terms of universal concepts regardless of what language it’s working in?”
The researchers found that LLMs do both. They asked Claude to translate simple sentences into multiple languages and tracked overlapping tokens it used during processing. Those shared tokens—that is, snippets of meaning—represented core, language-agnostic ideas like “smallness” or “oppositeness.” And using those two tokens in combination resulted in the representation of another universal concept meaning “largeness” (the opposite of small being large). The model uses these universal concepts before it ever translates them into a given language for the user.
This suggests that Claude can learn a concept like “smallness” in one language and then apply that knowledge when speaking another language with no additional training, Batson says. Studying how the model shares what it knows across contexts is important to understanding the way it reasons about questions in many different domains.
LLMs can plan and improvise
Claude isn’t just thinking about the next logical word to generate, it also has the ability to think “ahead.” When prompted by the research team to write poetry, Claude indeed incorporated rhyme schemes into its processing patterns. For example, after a line ended with “grab it,” Claude selected words in the following line that would nicely set up the use of “rabbit” as a conclusion.
“Someone on my team found that right at the end of this line, after ‘grab it,’ before it even started writing the next line, it was thinking about a rabbit,” Batson says. The researchers then intervened at that very point in the process, inserting either a new rhyme scheme or a new ending word, and Claude shifted its plan accordingly, picking a new verbal path to get to a rhyme that made sense.
Batson says the poetry observation is one of his favorites because it gives a relatively clean look at a specific part of the LLM reasoning through a problem, and because it proves that his team’s observation tools (e.g., the AI microscope) work.
The poetry study highlights just how much work remains to be done. The element of the LLM that’s activated by the poetry-related generation is very small relative to the full universe of tasks the model can do. Industry researchers are taking snapshots, in the way a neuroscientist might study the way one area of the human hippocampus converts short-term memories into long-term ones.
“Exploring that crazy space is like a bit of an adventure every time, and so we actually just needed tools to even see how things were connected and try ideas and move around,” Batson says. “So we kind of have this investigation phase after we’ve built the microscope and we’re looking at something [and saying] ‘Oh, okay, what is that part?’ and ‘What’s that part?’ and ‘What’s this thing over here?’”
But assuming that AI companies continue funding and prioritizing mechanistic interpretability research, the snapshots will pan wider and begin to interconnect, giving a broader understanding of why LLMs do what they do. A better understanding of those patterns could give the industry a better understanding of the real risks the systems might pose, as well as better ways to “steer” the systems toward safe and benevolent behavior.
Batson points out that we may develop more trust for AI systems over time by gaining more experience with their outputs. He adds, however, that he’d be “a heck of a lot more comfortable if we also understand what’s going on [inside].”
Войдите, чтобы добавить комментарий
Другие сообщения в этой группе


Rumors of a Tumblr comeback have been bubbling for a couple of years—think a pair of Doc Martens here, a splash of pastel hair dye there. Now, Gen Z is embracing the platform as a refuge from an i

You can’t talk about the manosphere without mentioning Andrew Tate. The British-American influencer and former professional kickboxer built his platform by promoting misogynistic ideas—claiming wo
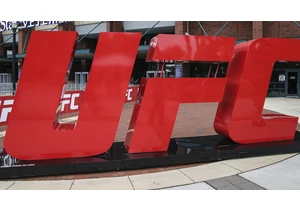
UFC is joining up with Facebook’s parent company

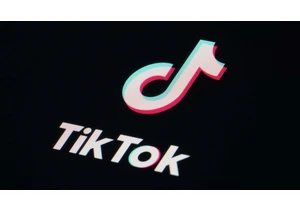
As the deadline to strike a deal over TikTok approaches this week, President Donald Trump has signaled that he is confident his administrat
